Extending Asset Life with Predictive Maintenance
Background
The two major technological advancements in railways are related to improving safety and introduction of predictive maintenance. In the last few years, railways have started to take the holistic cost of railway maintenance as a parameter for decision making and planning. Effective asset management with a view to increasing its life in service has become a very important factor. All railway components have an estimated lifespan based on their material properties and environmental and load conditions subjected to them. Predictive maintenance is built around making regular inspections of their condition, recognising when maintenance and repairs are needed, and identifying root causes behind accelerated aging or breakdowns such that these can be eliminated to increase asset life.
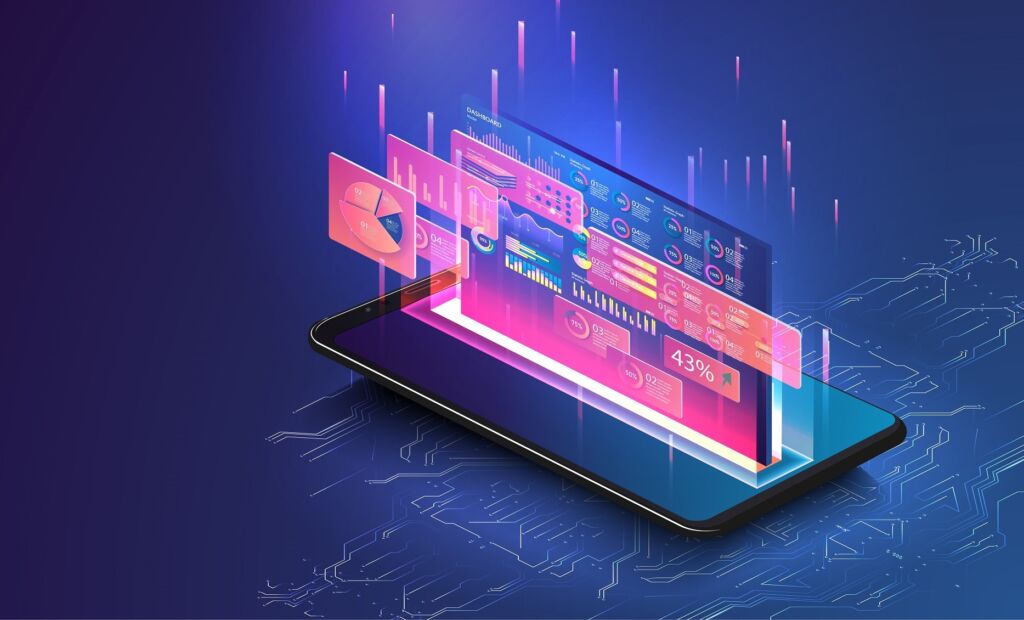
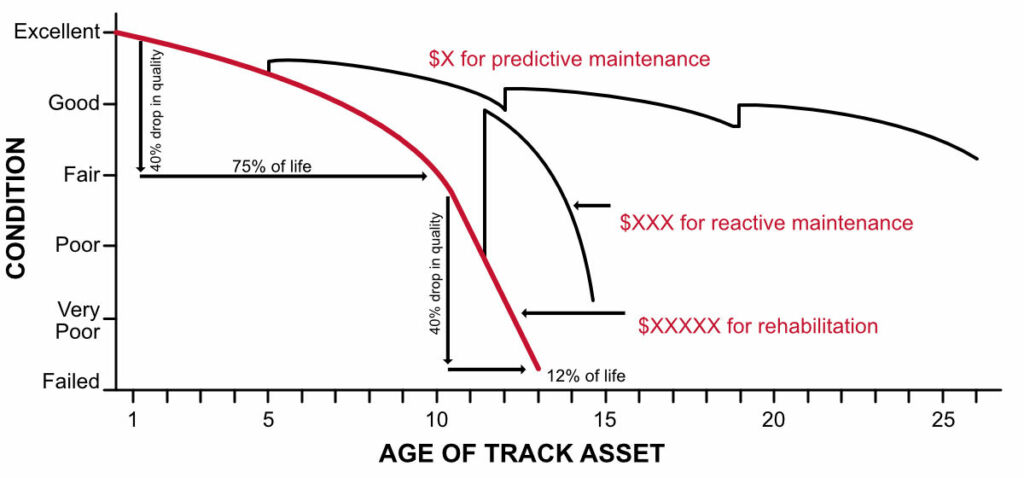
The regular passage of loads on a rail track can cause rail fatigue and damage the wheel. Internal stresses within the rail can create a range of defects both on the surface and internally which have the potential to break the rail and derail the moving vehicle. The track under load experiences a range of forces both longitudinally and laterally, as well as creates reaction forces on the wheel. It is well-known that preventive maintenance costs less money as shown in the figure to the left and extends the life of the component the most. The trick is to constantly monitor the asset. Reactive maintenance costs a lot more, and poorly maintained assets that suddenly fail cost even more money because of their limited lifespan.
Cost of component failures
The cost of component failures must not be under-estimated. Rails, sleepers, train wheels, bearings and so on are used in large quantities and these are expensive items. The cost of failure can be very high especially if it leads to an accident. Otherwise, the cost of repairs and an extended out of service period, or loss of revenue because of track or train unavailability can be very high. In the following we take a simple example to illustrate the importance of predictive maintenance and highlight the costs incurred by premature failure of assets. We take a simple example of a 350km metro network, with 1560 kms of rail and 2280 rail cars. If appropriate predictive maintenance approach is adopted, e.g. performing rail grinding correctly, maintaining appropriate track geometry and load conditions for optimal rail wheel interface, and so on, rails and wheels will not damage each other and last close to their expected lifespan. Poorly monitored and maintained railtrack will lead to severe financial losses as explained below where the example is simplified to take material costs alone into account; in reality several other costs will add up to these figures.
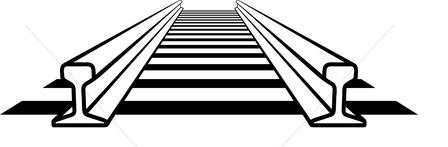
RAIL REPLACEMENTS COSTS FOR 350 KM METRO TRACK
Rail line rail cost ~ USD 866/MT, 45R
Entire network rail cost, double track ~ USD 1350 million
(1560Km of rail)
Investment into rail per year is USD 54 million /year at ideal life of 25 years
For each year of rail life reduced, customer loses USD 54 million
e.g. rail replaced after 15 years cost customer USD 90 million/year as opposed to USD 54 million
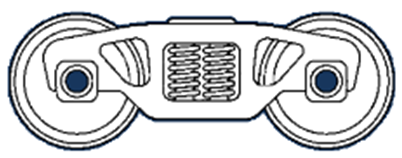
WHEEL REPLACEMENTS COSTS FOR A 350 KM METRO TRACK
Total rail cars 2280, total wheels 18240
Cost of each wheel USD 800, total wheel cost USD 14.6 million
Investment into wheels is nearly USD 1 million assuming a 15 year lifespan
For each year of wheel life reduced, customer loses USD 1 million
e.g. all wheels replaced after 7 years instead of 15 years cost USD 2 million/ year as opposed to USD 1 million/year
TrackVue + AI = Predictive Maintenance
Rail Vision TrackVue products are designed for regular monitoring of track and rolling stock assets. Regular inspections build up a history of asset health showing the rate of deterioration. TrackVue series Z, S, V and R products provide a wealth of raw measurement data which can be input into Artificial Intelligence based Machine Learning tools. Rail Vision Cloud Services provide a platform for such analytics to generate reports that can be used by customers to plan predictive maintenance. Some of the key technologies used by Rail Vision data analytics modules include:
- Multivariate prediction of component lifespan based on historic data showing condition deterioration and its material properties
- Track condition modelling using fuzzy data clustering
- Neural network based exception detection
- Understanding root cause behind specific issues, e.g. too frequent rail or wheel profiling by analysing rail wheel interface parameters