Smart Scanner Innovation
SafetyNET
Rail Vision has a prototype under trials in the UK for its SafetyNet product that automates the detection of explosives and dangerous objects in luggage when scanned with an x-ray scanner. In parallel, our collaboration with Todd Research is exploring the application of this technology to a wide variety of scanners. This industrial research projects trials a SafetyNet device for interpretation of x-ray images from security scanners for carry-on, hold luggage and parcels using automated image interpretation with deep learning technology. The system automatically determines the level of threat posed by the object under the scanner, and provides a safety net around the human operator decision making. The device output is integrated with existing scanners and used to alert the operator to the presence of threat items. The device is tested on dual energy x-ray equipment in laboratory and at customer sites. The device has universal appeal, integrating to a number of scanner models, making it a perfect option for retrofitting and new product line integration. The underlying algorithms and detection technology is based around the use of adaptive self-learning neural networks which use underlying principles of deep learning. Such systems learn from millions of images scanned and tested by human operators in an online setting. Once trained these are applied for threat detection to assist human decision making. As more data is scanned, these systems will have larger archives to learn from, and will continue to grow their intelligence over time. The systems are expected to markedly improve performance in terms of increased quality of threat detection, higher screening throughput and reduced false alarms and resulting delays. SafetyNet will be offered to potential customers as a combined hardware/software offering with a cloud backend to which the device will communicate data on system and operators, which can be used subsequently for delivering data services to customers to report on reducing the risk of visual fatigue and inadequate staff training. The target markets for such system will include stadiums, arenas, border control, ports, aviation, commercial head offices, data centres and defence applications.
Need for automation
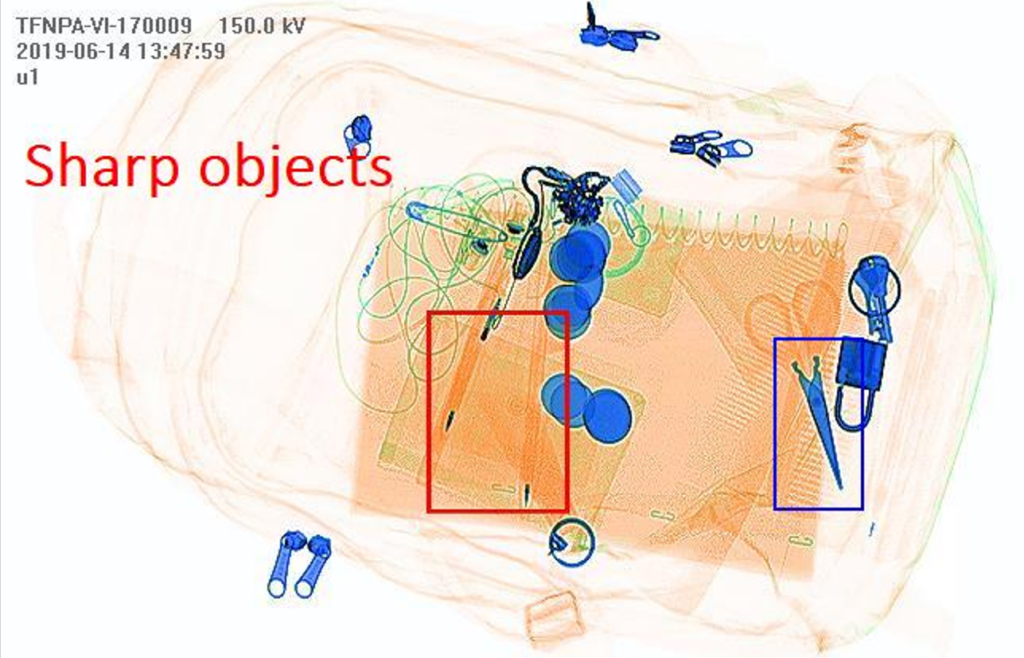
The existing luggage and parcels scanning practices at airports, train stations etc. could compromise security due to the inefficiencies of the existing 2D x-ray scanning technology and threats missed by human operators (screeners). Considering the limited time(~6sec/bag) given to the human operator to view and decide on a complex images, threat items may be missed. For high speed applications, < 2sec screening of parcels, most existing systems are too slow. Furthermore, delays at checkpoints related to false alarms with electrical goods/ cosmetics is a major cause of delay and frustration for all concerned. There is a compelling argument for bringing in new technology for substantially improving the accuracy and throughput of threat detection systems, integrating such technology with existing scanners, irrespective of scanner model, several of which have very limited level of AI based automation or suffer with poor performance issues, and delivering an adaptive system that can continue to improve over time.
Challenge
Ever-evolving threat of terrorist attacks in airports/trains and subway stations/embassies/government buildings/stadiums/museums/hotels require reformation to the global security screening outlook. Quick/precise identification of any suspicious objects/materials found inside/together with luggage and parcels in these critical infrastructure facilities demands innovative solutions. Existing screening practices with human operators suffer from visual fatigue and poor training, and for high speed applications insufficient decision time/image e.g. ~6sec/bag luggage scanners and < 2sec/parcel for logistics applications. Current screening processes are time consuming, require substantial manpower, require passengers to take out several personal items from baggage before screening causing delays, generating false alarms because of image scene complexity, and potentially failing to detect threats. Scanners quality and screening personnel is variable and no existing solution caters for their bespoke/diverse needs. Furthermore, there is a lack-of-technology and scanner plug-in devices that can address the quality of inspection across applications (luggage/parcels/vehicles/cargo).
Recently, leading scanner manufacturers (Smiths/Rapiscan/Nuctech/Adani/Synapse/Aurora/Astrophysics) have commercialised or are developing a range of analytics tools to assist scanner operators in guiding their attention to threat items and improving image quality. These tools are available with selected scanner models, proprietary, unpublished, heavily customised and vary significantly in terms of their benefits. Consequently, it would be a significant CAPEX cost for customers to replace all of their scanners with new models.
False positives in security screening remain a major headache; operators may often switch off such scanner functions if found to interfere/ cause delays. False positives often result because of how software for automated image interpretation is trained and embedded within scanners using template and rule based matching principles/techniques that often suffer greatly when the same object is visible with different perspectives, hidden, varies in size/appearance, and may not be in fully assembled form. Existing scanners also fail to automatically recognise mobile phones, cosmetics, laptops, keyrings, and harmless other objects which people often forget, causing major delays. Recently introduction of 3D CT technology by scanning manufacturers is an attempt to improve their detection capability however it is very expensive > £250K, has a limited roll-out, and lacks proven record for improving safety.
Building upon existing capability of successful use of deep learning technologies for automated railway track and train defect detection using high speed image analytics; Rail Vision (RVEL) in collaboration with Todd Research (TRL) are trialling its SafetyNet system which uses self-learning Online Deep Learning technology, a significantly superior approach to threat detection.